Alex Brady, an undergraduate physics student in the College of Science and Mathematics at Augusta University, recently won an outstanding presentation award for his research at the American Physical Society conference.
The award is only given to three student presentations during each student session. Altogether, more than 10,000 participants gathered virtually and in person to show off their research and attend seminars during the March 6 conference.
“I was surprised. There were so many amazing presentations that I attended,” said Brady. “I thought, ‘Wow, this is amazing research. There’s no way I’m going to be among the top presenters.’”
Trinanjan Datta, PhD, a professor in the Department of Chemistry and Physics, presented with Brady at the conference. Their research involves code that Brady developed for a machine-learning algorithm, LASSO, which simulates the wave patterns of a one-dimensional Heisenberg Chain, a model used to visualize magnetic systems.
The purpose of this algorithm is to uncover patterns in data that have been obscured by noise masking the data, which would look like dots scattered over a wavy line. The research involved adding synthetic noise to simulate impurities into an accurate magnetic system, then applying LASSO to remove the noise and reveal the original pattern underneath.
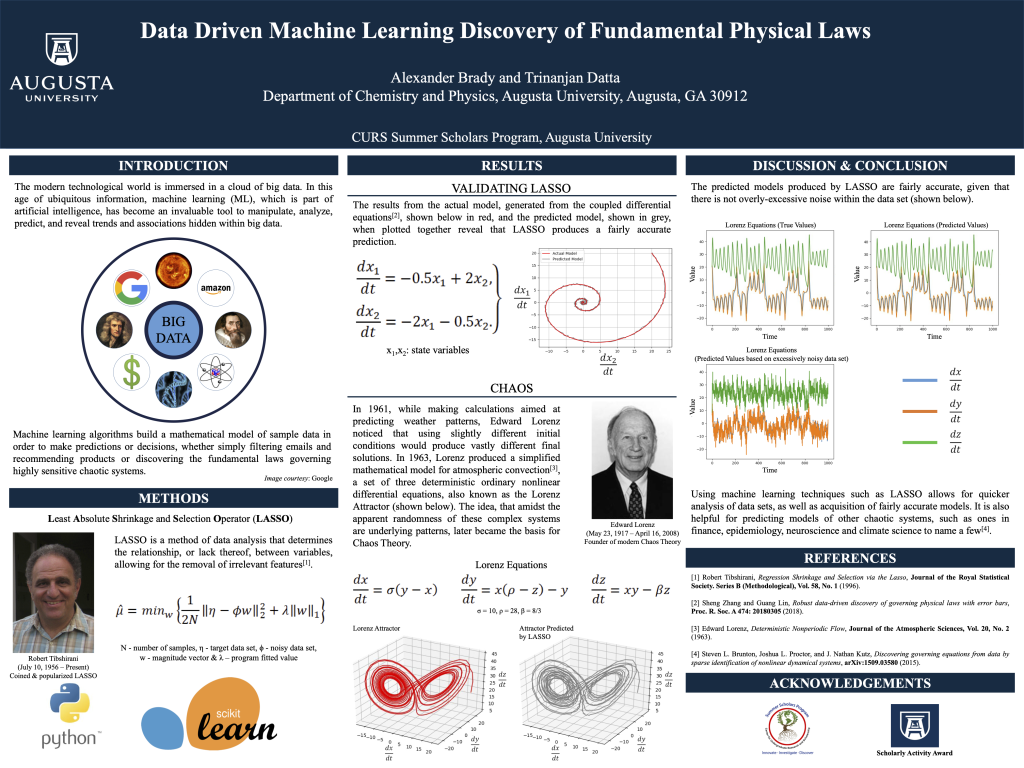
“For example, you look out in a cloudy sky, there’s nothing there. Then you apply a filter on it and there’s a spaceship out there,” said Datta. “When an experimentalist collects data, you have to be certain. Is this what you predicted?”
In condensed matter physics, the study of materials in their solid forms, LASSO is particularly useful. Even if a substance is made in a lab, it cannot be completely pure. These impurities can create noise in data, which LASSO fixes.
The potential applications for such an algorithm are not limited to condensed matter physics. Brady hopes to apply it to astronomy and astrophysics, and he collaborated with Datta to learn the coding necessary to apply machine learning to planetary movements.
“It can be applied to just about any set of big data that has noise,” said Brady. “Whether that noise is from, say, an economic scenario from random trends that occur — which may not be the general trend of the system — or back to astronomy and astrophysics, it could be the motion of an interplanetary system.”